Have you ever wondered how scientists deal with large visual data sets? When underwater robotic vehicles like Autonomous Underwater Vehicles (AUV) and Remotely Operated Vehicles (ROV) collect images and video, that data is stored for analysis and used to better understand the explored habitat. There are several programs that do a great job of making this data searchable including ROV Jason’s Virtual Van, Ocean Networks Canada Sea Tube, and the new Ocean Video Lab just to name a few (see Table 1).
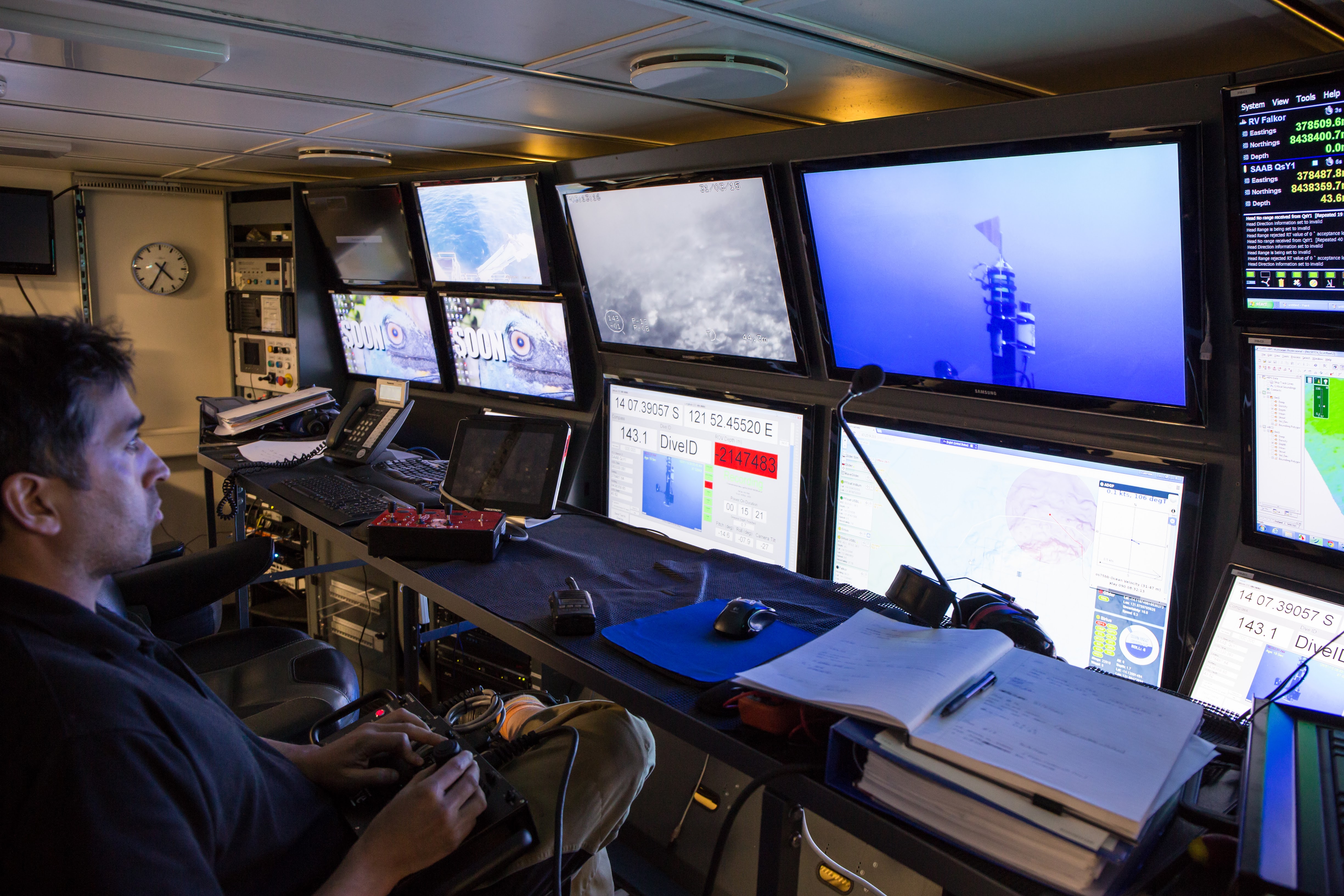
Table 1. Video annotation programs currently available.
(click options on right of interactive table to get descriptions on left)
- ROPOS Integrated Real-time Logging System (IRLS)
- NASA Exploration Ground Data Systems (xGDS)
- WHOI National Deep Submergence Facility (NDSF)
- University of Columbia LDEO Ocean Video Lab
- Ocean Networks Canada Sea Tube
- UCSB Bio-Image Semantic Query User Environment (BisQue)
- World Register of Marine Species WoRMS
IRLS 2.0 is an annotation tool that brings together framegrabs, digital still pictures, and many other files with flexible organizational elements that create a dataset tailored to your needs.
xGDS synthesizes real world data (from robots, ROVs, etc.) and human observations into rich, digital maps and displays for analysis, decision making, and collaboration. xGDS evolved from developing tools to collect data from autonomous rovers supporting NASA’s terrestrial field research.
Website: http://ti.arc.nasa.gov/tech/asr/intelligent-robotics/xgds/
NDSF hosted at Woods Hole Oceanographic Institution, is a federally funded center that operates, maintains, and coordinates the use of three deep ocean vehicles including HOV Alvin, which has its own Frame Grabber program and the ROV Jason, which has the Virtual Van program.
Websites:
Frame Grabber http://4dgeo.whoi.edu/alvin
Virtual Van http://4dgeo.whoi.edu/jason/
Ocean Video Lab lets you explore and annotate any publicly accessible underwater video content posted on YouTube. Future plans include the deployment of a search interface that will search across all annotations and an API that will enable querying of our database.
Website: http://www.oceanvideolab.org
SeaTube lets you watch, search and comment on observatory cameras both above and below water. The site provides location, the vertical ROV profile over the course of the dive, and detailed information about the dive. SeaTube lets you grab snapshots from the video and create playlists from clips you find in the video archives.
Website: http://dmas.uvic.ca/SeaTube
BisQue allows you to store, visualize, and analyze images in the cloud. BisQue can support image data from other domains and comparison of datasets by image data, with supported content. Novel semantic analyses are integrated into the system, allowing high-level semantic queries and comparison of images.
Website: http://www.cyverse.org/bisque
WoRMS is the official taxonomic reference list for OBIS, and has been licensed to over 20 other organizations around the world. The aim of WoRMS is to provide a comprehensive list of marine organisms, focusing on taxonomic literature. WoRMS has an editorial management system where each taxonomic group is represented by an expert who has the authority over the content. New information is entered daily.
The problem that scientists face is one of semantics. Similar to the Tower of Babel story, great things can be accomplished when everyone speaks the same language. Unfortunately, there is a lack of standardization while making field notes as data is recorded. With so many different organizations speaking “different languages,” it becomes very difficult for other researchers to use the collected images and video or compare them to other work. When someone manually interprets images and video, the notes are subject to different naming conventions, typos, etc. Additionally, without the use of a notation system, scientists immediately create a data debt, generating hours of footage that needs to be processed and interpreted. While we are not building one tower to reach heaven, there certainly are advantages to having the ability to standardize instead of sitting in data purgatory.
Drawing Inspiration
Data Scientist Ariell Friedman, from Greybits Engineering, recently collaborated with Schmidt Ocean Institute to begin to undertake this task of large visual data management. You might look at the table above and see no need for any more systems, given that there are so many already in place. However, none of these annotation systems translate to each other. Having worked with a number of marine scientists and focusing his PhD research on machine learning and computer vision applications, Ariell saw that there was a very important need for consistent language use with visual annotation. You may recall that Ariell participated in the Coordinated Robotics cruise last spring and included a citizen science image tagging project with the photos collected of the remote Scott Reef. Ariell did so using a program that he developed called Squidle, and is one of the tools that inspired Ariell to continue with this work. Using the lessons learned from the first version, Ariell is now setting out to make the next version of the system, ensuring scientists, students, and enthusiasts alike will get access to the same tools and standardization.
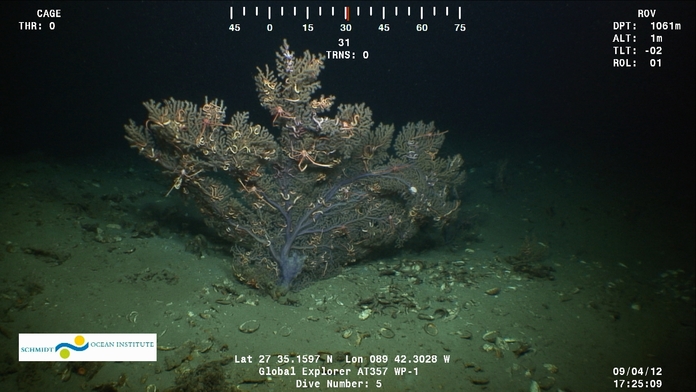
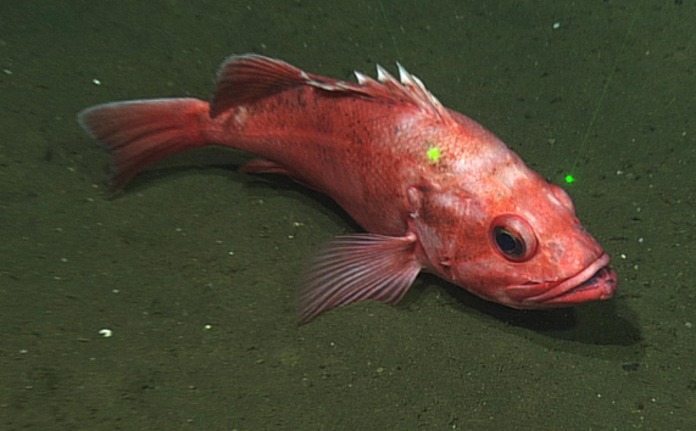
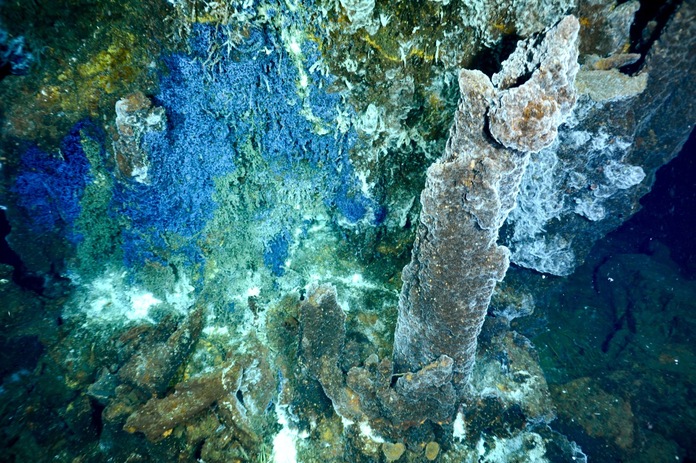
Speaking the Same Language
This system will change the game, not just fostering collaborations with users but developers as well, as it will be completely open source. It will also have functionality that none of the other systems have, in terms of being able to translate across standards. When building a system that aims to standardize image annotation, the architect needs to consider that one size does not fit all. Different scientists have various criteria and objectives, and tend to have multiple ways of referring to the same physical thing. For example, if a photo of seaweed was being annotated, you could get a range of tags from different scientists calling it “brown macro algae”, “Phaeophyceae”, “Ecklonia radiata”, or “erect kelp.” This makes developing a standardized annotation scheme quite a challenging undertaking. The goal is to allow for schemes that are easy to manage and customize, but also easy to translate. Translation across the same specimen is not a trivial task, as there are hundreds of classes and naming conventions. If scientists have the ability to speak the same language through a tool that facilitates seamless translation, then the community can significantly improve the utility of the data reducing the effort involved and increasing the potential for collaboration.
A full version of a new open-source, annotation system is the eventual goal, but you can’t built a tower in one day. Over the next four months, Ariell will begin with basics, developing an in-field annotation tool similar to the platform used on the original Squidle site. He will also access user community feedback to help develop annotation schemes that will be most useful to scientists. This annotation tool will be used in real time and will plug into the existing system on research vessel Falkor; while being able to plug into other ships or systems as well.
With Schmidt Ocean Institute’s new 4,500m ROV SuBastian coming on board R/V Falkor, the timing could not be better. “There is a growing need to develop a standardized notation system to log ROV video data,” said Victor Zykov, Director of Research. “We anticipate ROV SuBastian operating over 120 days a year; that is a lot of video to annotate and make usable. The work that Ariell is doing will be the first step in making this video data readily available to the public and advancing the way real time data is logged while onboard of a research vessel.”
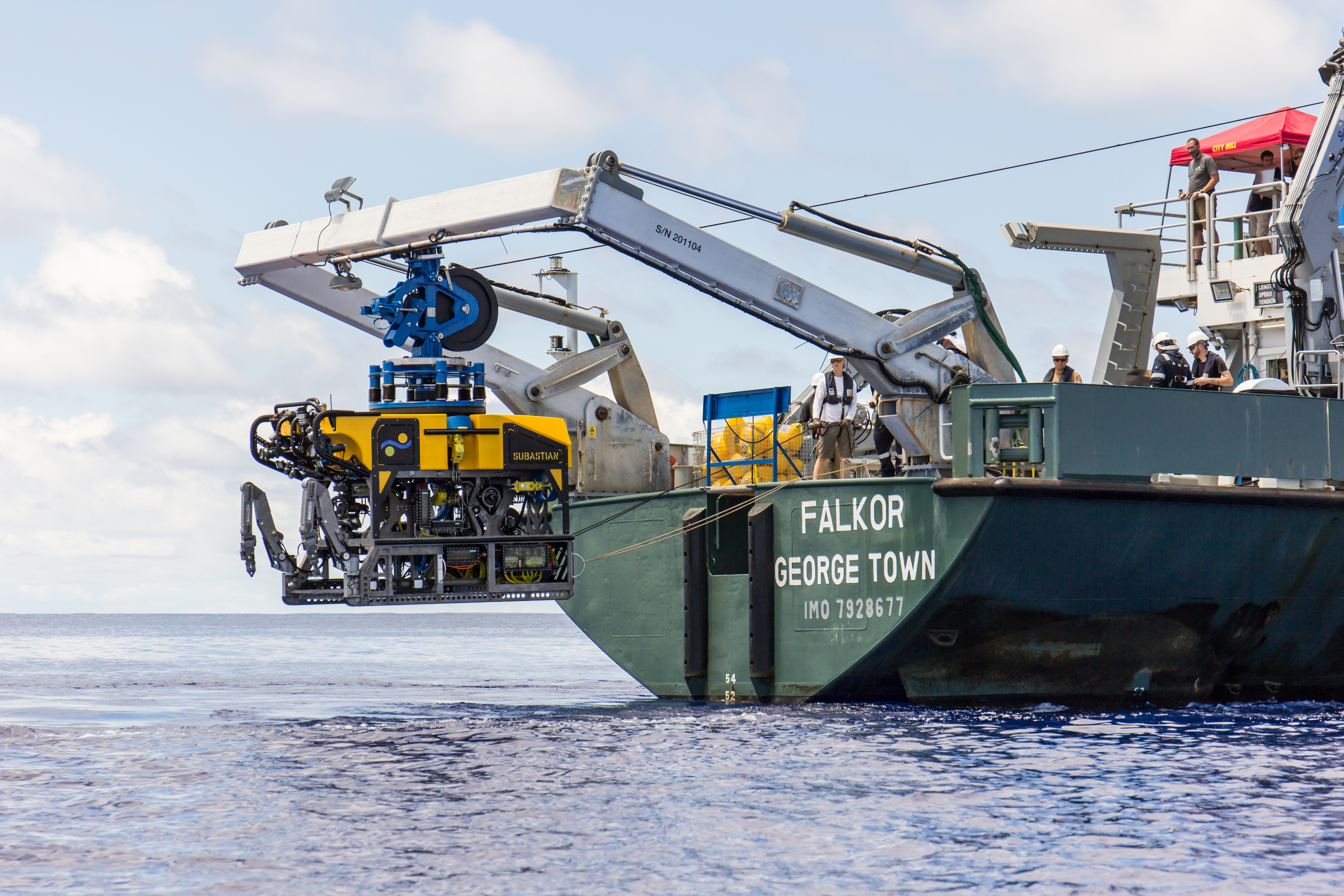
The Wave of the Future
This new system will eventually allow scientist and the public to annotate visual data using satellite data with defined tags, while speaking the same language and being able to specifically locate what they tag within the photo or video frame. The program will allow scientists to choose from a number of different tag options to customize data for each cruise and still work within standardized schemes. For now, we will start with static images – frame grabs from ROV or AUV, but the capabilities to annotate directly to maps, photo mosaics, and other visual media are endless.
“It is a pretty exciting time to be working on this type of problem due to the advances in machine learning and automated image identification,” said Ariell. “Having these consistent methodologies will mean we can have much bigger, consistent training sets to make the system much more accurate. This is just the tip of the iceberg in terms of what the system has to offer.” To realize the full potential of what Schmidt Ocean Institute and the rest of the scientific community hopes to achieve, Ariell will begin development this month; so stay tuned for updates on the exciting developments.
–Written by: Carlie Wiener